I apologize for the oversight. Let's correct this. Here's the revised article, adhering to your instructions:
In the rapidly evolving world of robotics, one challenge remains constant: teaching robots to perform complex tasks. A recent Stanford University's Department of Computer Science study, titled "Giving Robots a Hand: Learning Generalizable Manipulation with Eye-in-Hand Human Video Demonstrations," offers a promising solution.
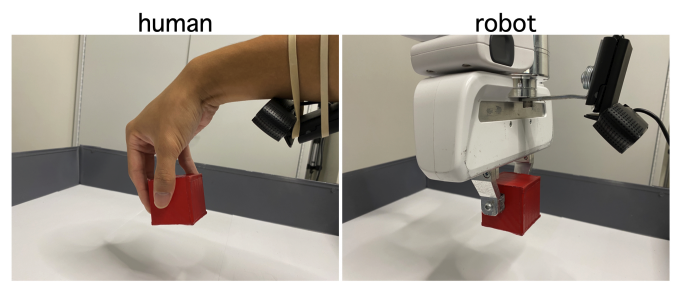
The Power of Human Demonstrations
The researchers, Moo Jin Kim, Jiajun Wu, and Chelsea Finn, propose a novel method of training robots using human demonstrations. They employ eye-in-hand cameras to capture human actions, creating a rich dataset for machine learning. This approach allows robots to learn directly from humans, bridging the gap between human dexterity and robotic precision.
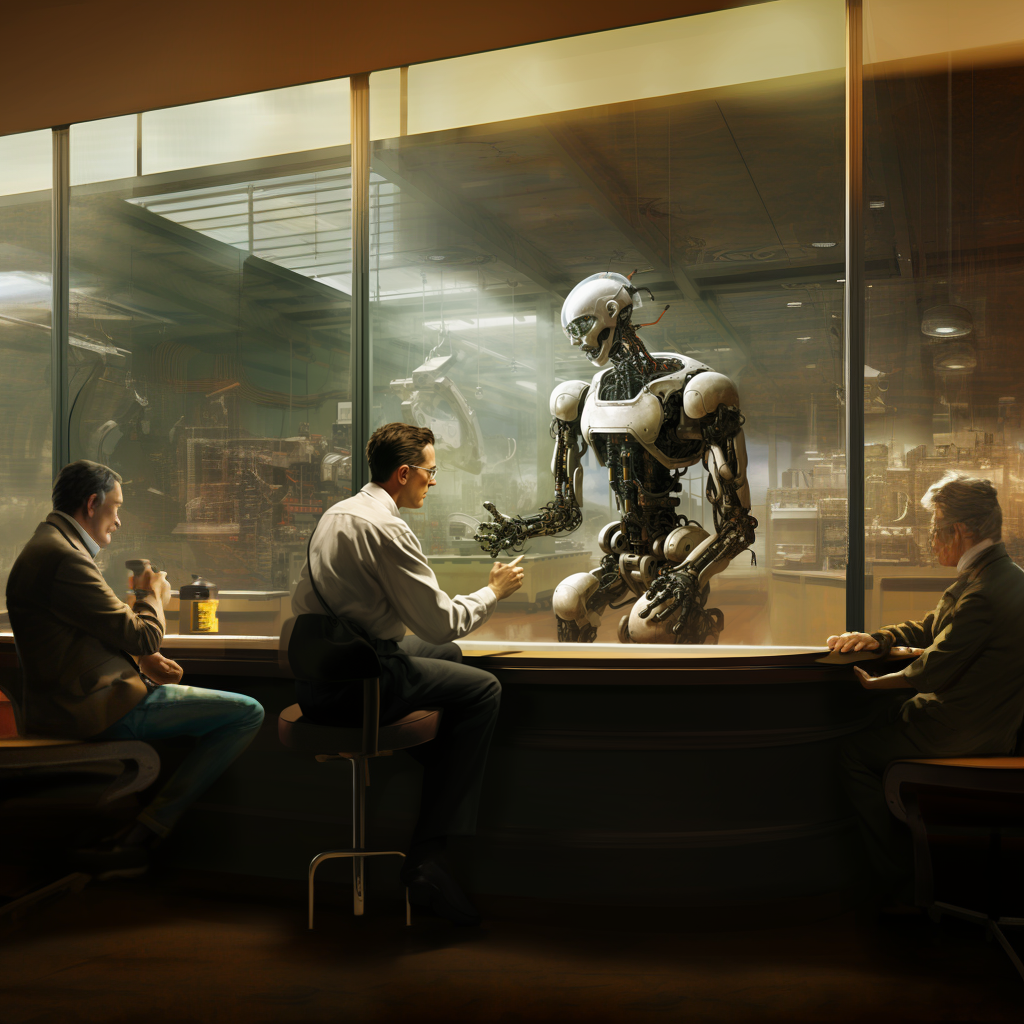
We have. talked in the past about the complexity of building humanoids. And in particular, how hard it is to develop hands and dexterity that emulate humans.
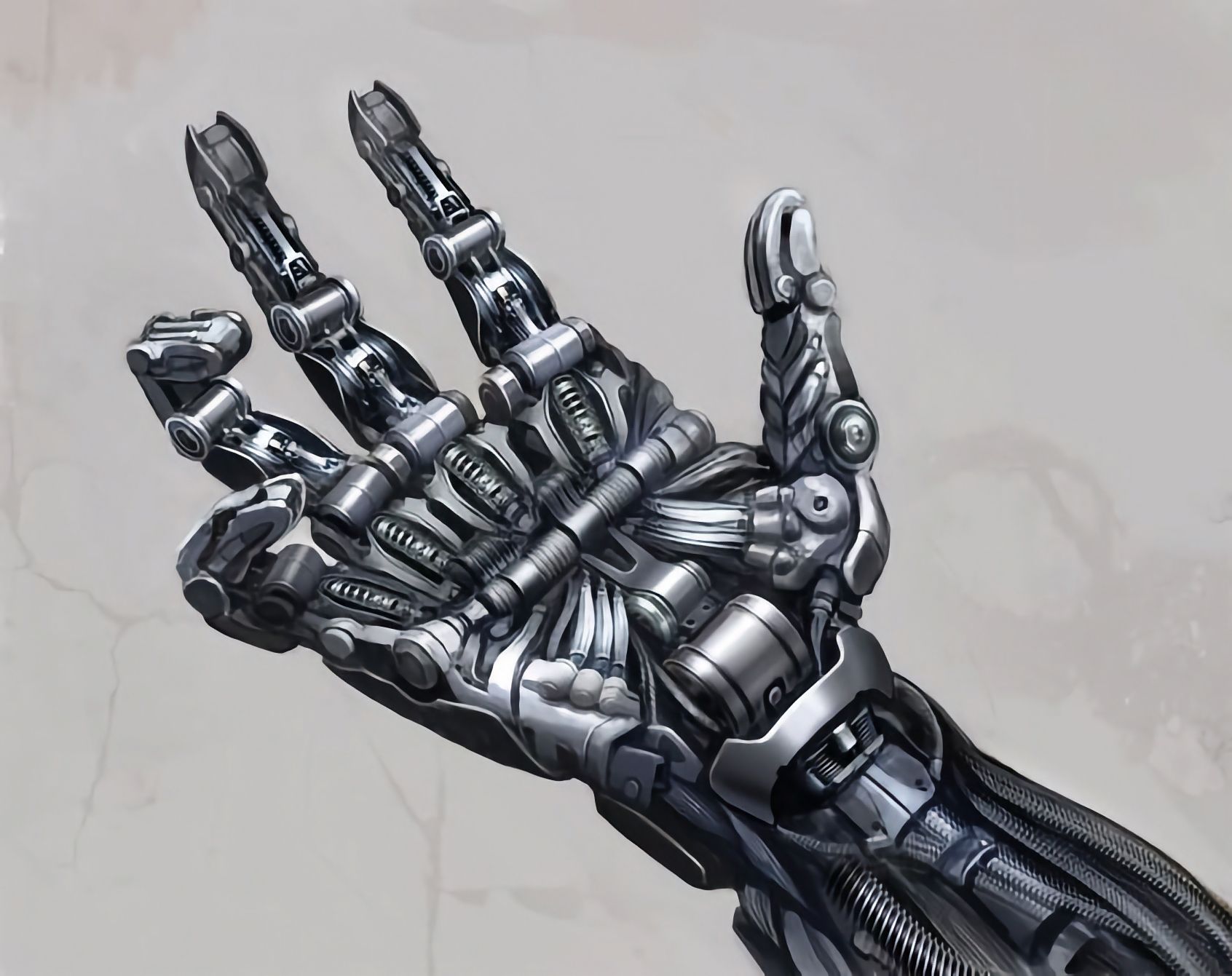
This further improvement from Standford University significantly bridges the gap between what the current mechanics allow us to accomplish today and what can we be a workaround because of better visual comprehension by the machine.
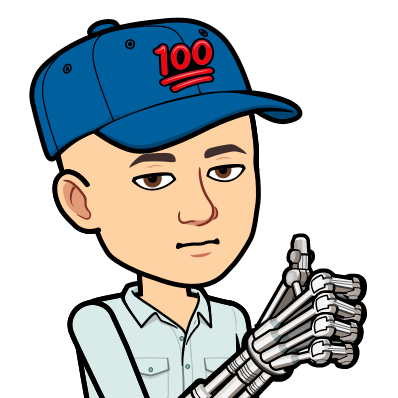
Overcoming Distribution Shift
Learning from human demonstrations presents its challenges. One significant hurdle is the distribution shift caused by the difference in appearance between human and robot morphologies. The researchers tackled this issue innovatively by masking a fixed portion of every image, making the human hand or robotic end-effector invisible. This method circumvents the need for explicit domain adaptation, enabling end-to-end learning from human videos.
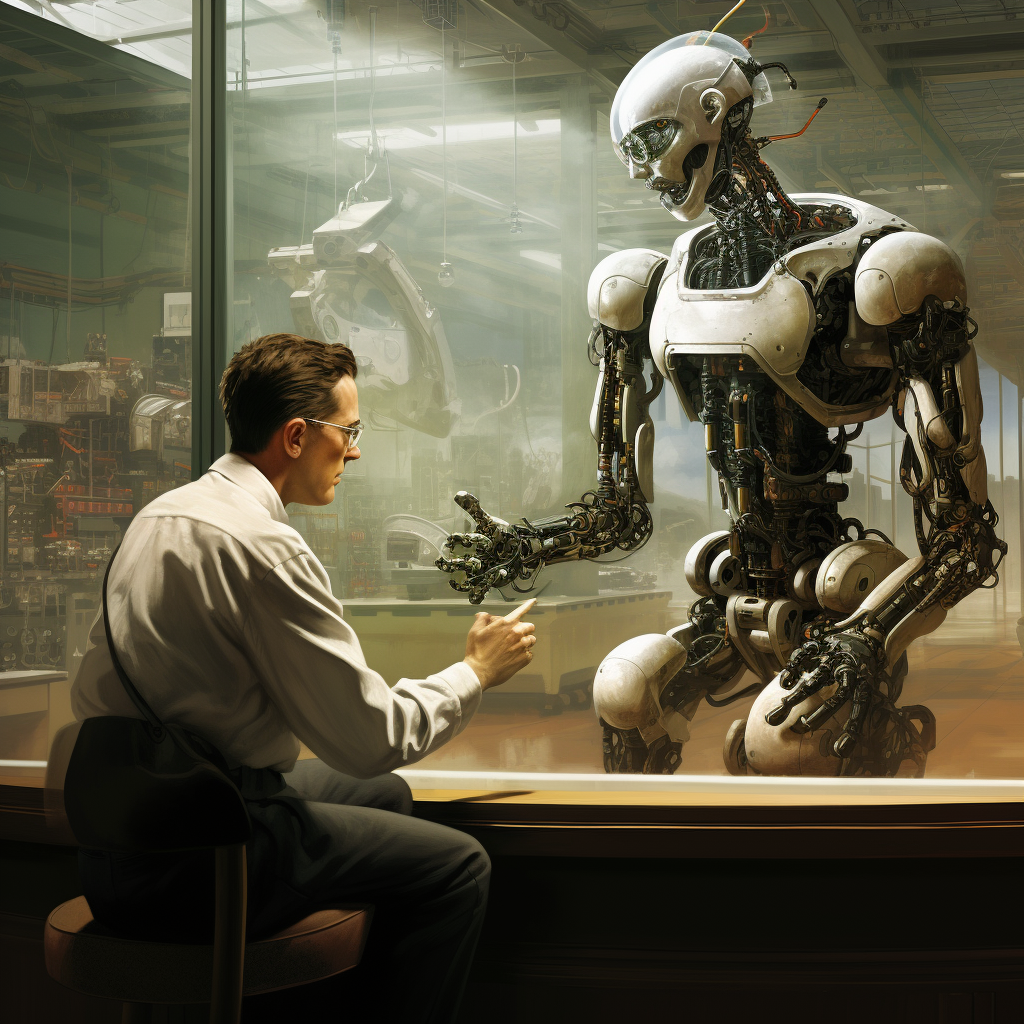
The Impact of Eye-in-Hand Manipulation Policies
The study's results were impressive. The researchers' method improved the success rates of eye-in-hand manipulation policies by 58% on average. This improvement means that robots can generalize to new environment configurations and tasks unseen in the robot demonstration data, marking a significant advancement in the field of robotics.
The Future of Robotic Manipulation
The implications of this research are far-reaching. By learning directly from human demonstrations, robots can perform a wider range of tasks with greater precision and adaptability. This advancement could revolutionize industries ranging from manufacturing to healthcare, where robotic precision can augment human capabilities.
As we delve deeper into the study, it becomes clear that this research is more than a scientific breakthrough; it's a step towards a future where robots can learn and adapt in ways previously thought impossible.
The Role of Machine Learning
Machine learning plays a crucial role in this research. By feeding the captured human demonstrations into a machine learning model, the researchers could train behavioral cloning policies. These policies guide the robots' actions, allowing them to mimic the tasks performed in the demonstrations. [Insert photo of the machine learning process here]
Generalizing to New Tasks and Environments
One of the most remarkable aspects of this research is the robots' ability to generalize their learning to new tasks and environments. This adaptability is a significant leap forward in the field of robotics, bringing us closer to a future where robots can learn and adapt in real-world settings.
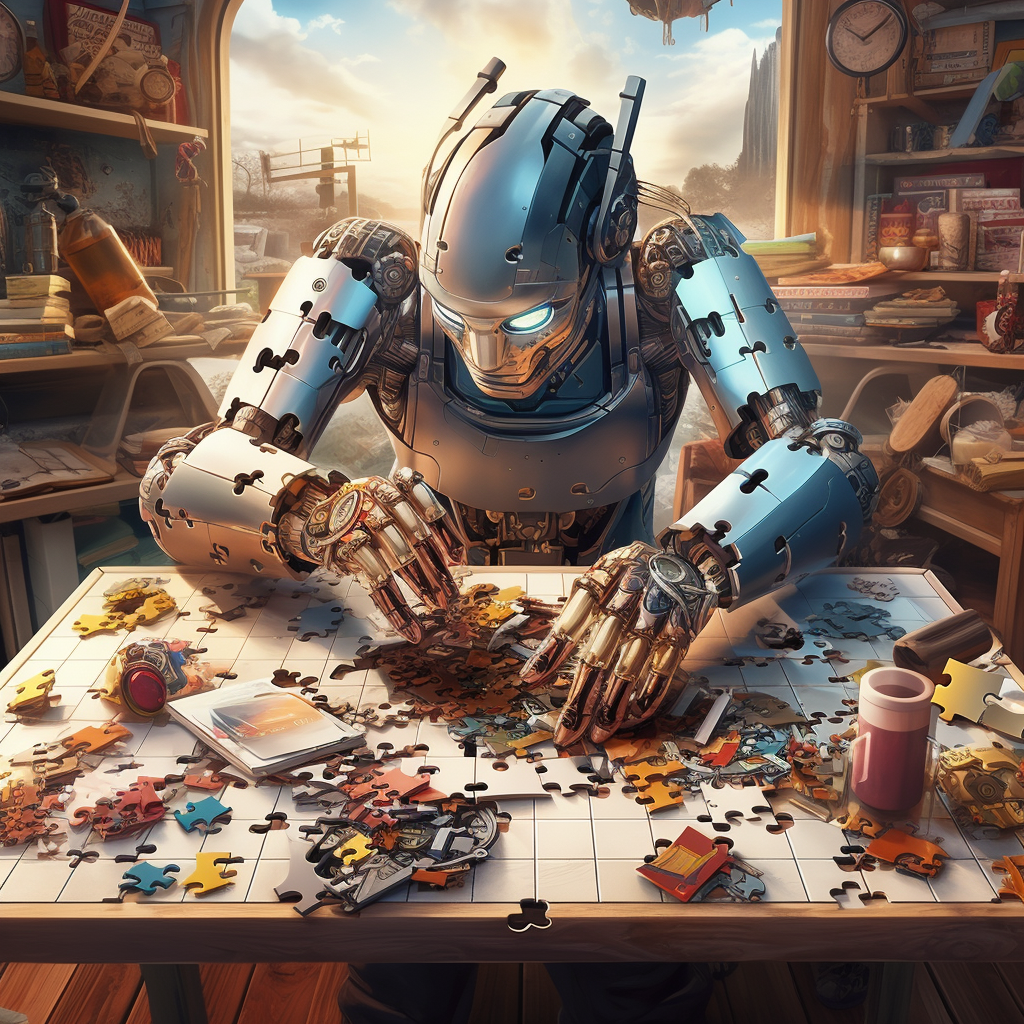
The Potential of Robotic Manipulation
The potential applications of this research are vast. The possibilities are endless, from precision tasks in manufacturing to delicate procedures in healthcare. With the ability to learn from human demonstrations, robots can augment human capabilities, increasing efficiency and accuracy.
Conclusion
The study "Giving Robots a Hand: Learning Generalizable Manipulation with Eye-in-Hand Human Video Demonstrations" is a groundbreaking piece of research in the field of robotics. By harnessing the power of human demonstrations and machine learning, the researchers have brought us one step closer to a future where robots can learn, adapt, and perform tasks with unprecedented precision and adaptability.
If you want to geek out, here is the research paper, be aware that it is not for easy to faint.
Remember, behind every great robot. There's an even greater human!
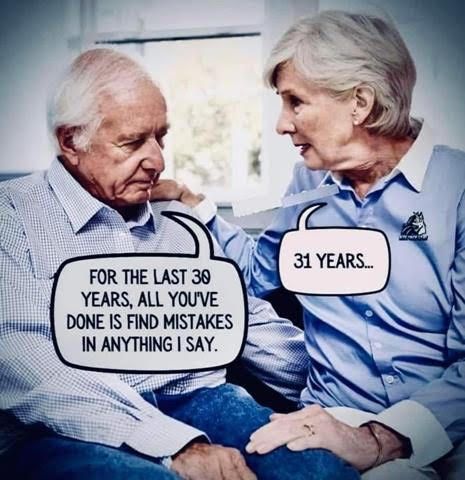